|
PointNet++是经典的三维点云分类/分割网络PointNet的续作,通过增加多层级多尺度的特征提取/聚合,来更好地处理点云密度不均匀的情况。
论文地址:PointNet++: Deep Hierarchical Feature Learning on Point Sets in a Metric Space
本文对PointNet++的源码进行研读,适合于已经读过论文或者对文章内容有一定了解的同学。
本文研读的代码主要参考论文作者的Tensorflow版本。
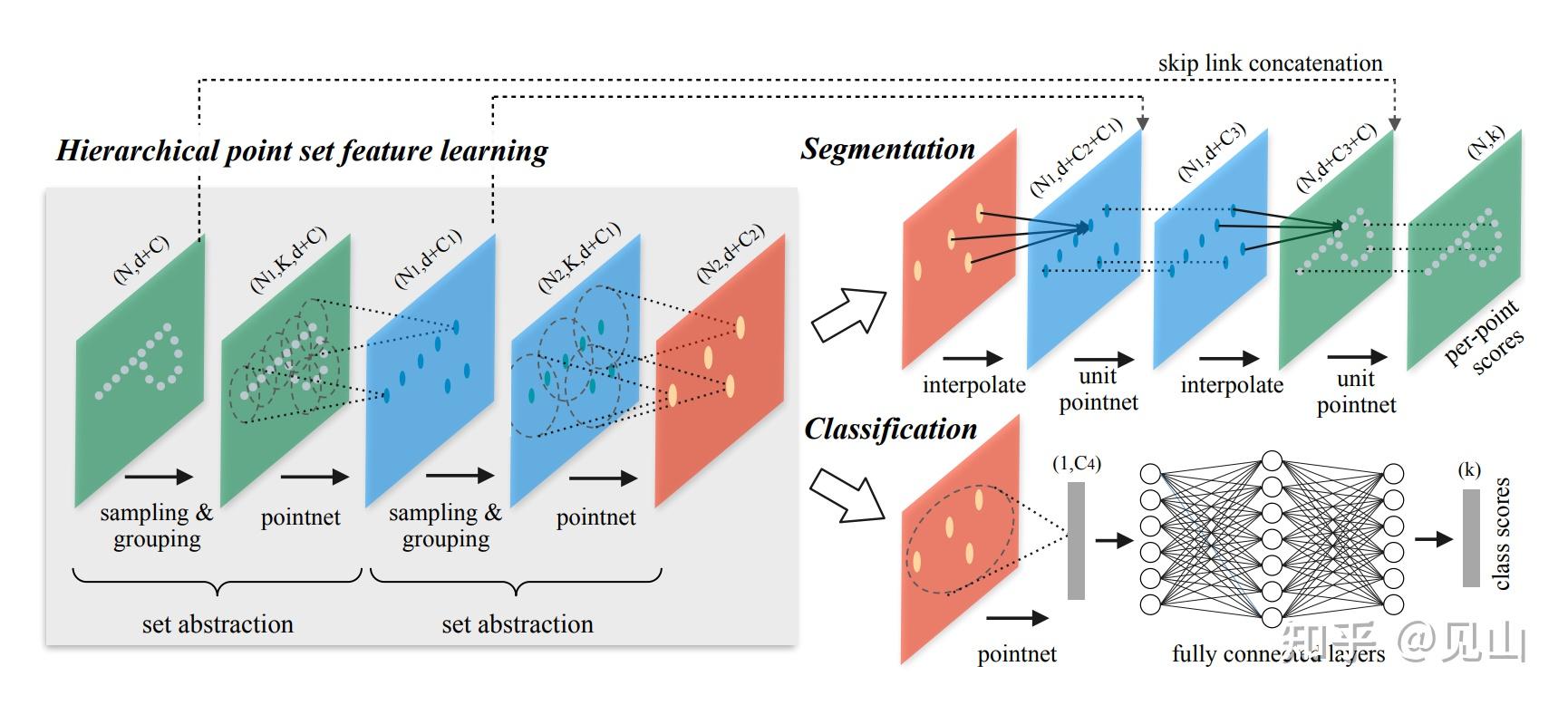
PointNet++的三个功能:分类(Classification)、语义分割(Semantic Segmantation)、部件分割(Part Segmentation),对应于三个不同的网络,但其核心都是Set Abstraction (SA) Module。这个模块对点云进行降采样并提取采样后的点的邻域特征,从而实现不同层级、不同尺度的特征提取与识别。通过多个SA模块的级联,可以同时兼顾点云的局部和全局信息。
以SSG(Single-scale grouping) Classification为例:
def get_model(point_cloud, is_training, bn_decay=None):
""" Classification PointNet, input is BxNx3, output Bx40 """
batch_size = point_cloud.get_shape()[0].value
num_point = point_cloud.get_shape()[1].value
end_points = {}
l0_xyz = point_cloud
l0_points = None
end_points['l0_xyz'] = l0_xyz
# Set abstraction layers
# Note: When using NCHW for layer 2, we see increased GPU memory usage (in TF1.4).
# So we only use NCHW for layer 1 until this issue can be resolved.
l1_xyz, l1_points, l1_indices = pointnet_sa_module(l0_xyz, l0_points, npoint=512, radius=0.2, nsample=32, mlp=[64,64,128], mlp2=None, group_all=False, is_training=is_training, bn_decay=bn_decay, scope='layer1', use_nchw=True)
l2_xyz, l2_points, l2_indices = pointnet_sa_module(l1_xyz, l1_points, npoint=128, radius=0.4, nsample=64, mlp=[128,128,256], mlp2=None, group_all=False, is_training=is_training, bn_decay=bn_decay, scope='layer2')
l3_xyz, l3_points, l3_indices = pointnet_sa_module(l2_xyz, l2_points, npoint=None, radius=None, nsample=None, mlp=[256,512,1024], mlp2=None, group_all=True, is_training=is_training, bn_decay=bn_decay, scope='layer3')
# Fully connected layers
net = tf.reshape(l3_points, [batch_size, -1])
net = tf_util.fully_connected(net, 512, bn=True, is_training=is_training, scope='fc1', bn_decay=bn_decay)
net = tf_util.dropout(net, keep_prob=0.5, is_training=is_training, scope='dp1')
net = tf_util.fully_connected(net, 256, bn=True, is_training=is_training, scope='fc2', bn_decay=bn_decay)
net = tf_util.dropout(net, keep_prob=0.5, is_training=is_training, scope='dp2')
net = tf_util.fully_connected(net, 40, activation_fn=None, scope='fc3')
return net, end_points
- 输入的原始点云经过第一个SA后降采样为512个点,每个点的特征中携带了其附近32个点的局部结构信息;
- 第一个SA输出的点云再经过第二个SA后降采样为128个点,每个点的特征中携带了其附近64个点的局部结构信息;
- 第二个SA输出的点云经过第三个SA后降采样为1个点,其特征向量高达1024维,即携带有所有点的整体结构信息;
- 第三个SA输出的特征向量通过一些全连接层(即MLP)后映射到分类结果的OneHot编码。
可以看到SSG Classification的顶层结构是比较简洁清晰的,其中最核心的就是pointnet_sa_module,下面来看这个module的实现:
def pointnet_sa_module(xyz, points, npoint, radius, nsample, mlp, mlp2, group_all, is_training, bn_decay, scope, bn=True, pooling='max', knn=False, use_xyz=True, use_nchw=False):
''' PointNet Set Abstraction (SA) Module
Input:
xyz: (batch_size, ndataset, 3) TF tensor
points: (batch_size, ndataset, channel) TF tensor
npoint: int32 -- #points sampled in farthest point sampling
radius: float32 -- search radius in local region
nsample: int32 -- how many points in each local region
mlp: list of int32 -- output size for MLP on each point
mlp2: list of int32 -- output size for MLP on each region
group_all: bool -- group all points into one PC if set true, OVERRIDE
npoint, radius and nsample settings
use_xyz: bool, if True concat XYZ with local point features, otherwise just use point features
use_nchw: bool, if True, use NCHW data format for conv2d, which is usually faster than NHWC format
Return:
new_xyz: (batch_size, npoint, 3) TF tensor
new_points: (batch_size, npoint, mlp[-1] or mlp2[-1]) TF tensor
idx: (batch_size, npoint, nsample) int32 -- indices for local regions
'''
data_format = 'NCHW' if use_nchw else 'NHWC'
with tf.variable_scope(scope) as sc:
# Sample and Grouping
if group_all:
nsample = xyz.get_shape()[1].value
new_xyz, new_points, idx, grouped_xyz = sample_and_group_all(xyz, points, use_xyz)
else:
new_xyz, new_points, idx, grouped_xyz = sample_and_group(npoint, radius, nsample, xyz, points, knn, use_xyz)
# Point Feature Embedding
if use_nchw: new_points = tf.transpose(new_points, [0,3,1,2])
for i, num_out_channel in enumerate(mlp):
new_points = tf_util.conv2d(new_points, num_out_channel, [1,1],
padding='VALID', stride=[1,1],
bn=bn, is_training=is_training,
scope='conv%d'%(i), bn_decay=bn_decay,
data_format=data_format)
if use_nchw: new_points = tf.transpose(new_points, [0,2,3,1])
# Pooling in Local Regions
if pooling=='max':
new_points = tf.reduce_max(new_points, axis=[2], keep_dims=True, name='maxpool')
elif pooling=='avg':
new_points = tf.reduce_mean(new_points, axis=[2], keep_dims=True, name='avgpool')
elif pooling=='weighted_avg':
with tf.variable_scope('weighted_avg'):
dists = tf.norm(grouped_xyz,axis=-1,ord=2,keep_dims=True)
exp_dists = tf.exp(-dists * 5)
weights = exp_dists/tf.reduce_sum(exp_dists,axis=2,keep_dims=True) # (batch_size, npoint, nsample, 1)
new_points *= weights # (batch_size, npoint, nsample, mlp[-1])
new_points = tf.reduce_sum(new_points, axis=2, keep_dims=True)
elif pooling=='max_and_avg':
max_points = tf.reduce_max(new_points, axis=[2], keep_dims=True, name='maxpool')
avg_points = tf.reduce_mean(new_points, axis=[2], keep_dims=True, name='avgpool')
new_points = tf.concat([avg_points, max_points], axis=-1)
# [Optional] Further Processing
if mlp2 is not None:
if use_nchw: new_points = tf.transpose(new_points, [0,3,1,2])
for i, num_out_channel in enumerate(mlp2):
new_points = tf_util.conv2d(new_points, num_out_channel, [1,1],
padding='VALID', stride=[1,1],
bn=bn, is_training=is_training,
scope='conv_post_%d'%(i), bn_decay=bn_decay,
data_format=data_format)
if use_nchw: new_points = tf.transpose(new_points, [0,2,3,1])
new_points = tf.squeeze(new_points, [2]) # (batch_size, npoints, mlp2[-1])
return new_xyz, new_points, idx根据论文中的表述,我们先来理解一下这个module想做一件什么样的事。
While PointNet uses a single max pooling operation to aggregate the whole point set, our new architecture builds a hierarchical grouping of points and progressively abstract larger and larger local regions along the hierarchy. 鉴于PointNet只是对输入的点云集合全体进行简单的MaxPooling操作,PointNet++试图将点云集合进行层级化的分组,并将各组子集区域中的信息进行层层抽象。具体来看,分为两步:
- 将输入的点云集合分组,得到N个子集(group);(通过先采样中心点,再搜索近邻点来实现)
- 在每个子集上通过微型PointNet来提取信息,得到:一个centroid point来表征这个子集在空间中的位置,一个特征向量来表征这个子集的结构信息。
所以可以理解pointnet_sa_module的输入输出:
- 输入:分组前的每个点的位置(xyz),分组前的每个点的特征向量(points),分组的数目(npoint),搜索半径(radius),每个组的点云数量(nsample),微型PointNet的配置(mlp,mlp2);
- 输出:分组后的每个点的位置/每个组的中心(new_xyz),分组后的每个点的特征向量(new_points),分组后每个点对应组内成员索引(idx)。
理解了要做什么事,也就更容易理解代码本身。忽略掉一些格式转换的代码,很容易发现:
- 上述第一步“将点云分组”是通过sample_and_group/sample_and_group_all这两个封装函数实现来实现(sample_and_group_all是将所有点云分成1组的特殊版sample_and_group);
- 上述第二步应用微型PointNet则是将代码直接写在pointnet_sa_module内部,略去对性能影响不大的transformation操作,PointNet其实就是MLP+MaxPooling,结构简单清晰,在这里最后的信息聚合操作,除了MaxPooling以外,作者还提供了AveragePooling和WeightedAveragePooling可供选择。
往下看点云分组函数sample_and_group:
def sample_and_group(npoint, radius, nsample, xyz, points, knn=False, use_xyz=True):
'''
Input:
npoint: int32
radius: float32
nsample: int32
xyz: (batch_size, ndataset, 3) TF tensor
points: (batch_size, ndataset, channel) TF tensor, if None will just use xyz as points
knn: bool, if True use kNN instead of radius search
use_xyz: bool, if True concat XYZ with local point features, otherwise just use point features
Output:
new_xyz: (batch_size, npoint, 3) TF tensor
new_points: (batch_size, npoint, nsample, 3+channel) TF tensor
idx: (batch_size, npoint, nsample) TF tensor, indices of local points as in ndataset points
grouped_xyz: (batch_size, npoint, nsample, 3) TF tensor, normalized point XYZs
(subtracted by seed point XYZ) in local regions
'''
new_xyz = gather_point(xyz, farthest_point_sample(npoint, xyz)) # (batch_size, npoint, 3)
if knn:
_,idx = knn_point(nsample, xyz, new_xyz)
else:
idx, pts_cnt = query_ball_point(radius, nsample, xyz, new_xyz)
grouped_xyz = group_point(xyz, idx) # (batch_size, npoint, nsample, 3)
grouped_xyz -= tf.tile(tf.expand_dims(new_xyz, 2), [1,1,nsample,1]) # translation normalization
if points is not None:
grouped_points = group_point(points, idx) # (batch_size, npoint, nsample, channel)
if use_xyz:
new_points = tf.concat([grouped_xyz, grouped_points], axis=-1) # (batch_size, npoint, nample, 3+channel)
else:
new_points = grouped_points
else:
new_points = grouped_xyz
return new_xyz, new_points, idx, grouped_xyz这个函数的功能是:将输入的ndataset个点按空间距离分为npoint个组,每组nsample个点。
具体实现上,先通过farthest_point_sample找出这ndataset个点中相互分布最为稀疏的npoint个点,作为npoint个组的中心,然后这些中心附近寻找k近邻或者距离中心radius范围内最近的nsample个点,如果radius范围内不够nsample个点,则进行重采样来补全。
总之,这个函数输入ndataset个点,输出npoint个中心点,每个中心点一定对应原点云中的nsample个点。为了记录这npoint个组的位置,只需要记录npoint个中心点位置即可,但是后面还要通过微型PointNet来提取信息,所以每个原始点的特征向量都要保留。
可以将特征向量的维度理解成图像里的channel,那么输入的一个数据样本(代码里的points)可以理解成一张一维图片,其长度为ndataset;而输出的点云特征向量(代码里的new_points)则是将原来的一维图片变换成了一张二维图片,其尺寸为npoint*nsample,而channel不变。
这也就是为什么原版PointNet使用一维卷积,而后面的微型PointNet使用二维卷积的原因。因为PointNet中的卷积只是起到组合不同channel信息的作用,所以无论一维二维卷积,卷积核的大小都是1。
微型PointNet的代码比较简单,没有什么可讲的。
以上就是SSG版本的pointnet_sa_module,这里Single-scale的含义指的是其中的radius和nsample都是一个标量,即对于全体输入的点云采用单一的空间尺度来进行分组。
与SSG相对的,作者再论文中还提出的两种更复杂的算法:MSG(Multi-scale Grouping)和MRG(Multi-resolution Grouping),但代码中只实现了MSG。
def pointnet_sa_module_msg(xyz, points, npoint, radius_list, nsample_list, mlp_list, is_training, bn_decay, scope, bn=True, use_xyz=True, use_nchw=False):
''' PointNet Set Abstraction (SA) module with Multi-Scale Grouping (MSG)
Input:
xyz: (batch_size, ndataset, 3) TF tensor
points: (batch_size, ndataset, channel) TF tensor
npoint: int32 -- #points sampled in farthest point sampling
radius: list of float32 -- search radius in local region
nsample: list of int32 -- how many points in each local region
mlp: list of list of int32 -- output size for MLP on each point
use_xyz: bool, if True concat XYZ with local point features, otherwise just use point features
use_nchw: bool, if True, use NCHW data format for conv2d, which is usually faster than NHWC format
Return:
new_xyz: (batch_size, npoint, 3) TF tensor
new_points: (batch_size, npoint, \sum_k{mlp[k][-1]}) TF tensor
'''
data_format = 'NCHW' if use_nchw else 'NHWC'
with tf.variable_scope(scope) as sc:
new_xyz = gather_point(xyz, farthest_point_sample(npoint, xyz))
new_points_list = []
for i in range(len(radius_list)):
radius = radius_list
nsample = nsample_list
idx, pts_cnt = query_ball_point(radius, nsample, xyz, new_xyz)
grouped_xyz = group_point(xyz, idx)
grouped_xyz -= tf.tile(tf.expand_dims(new_xyz, 2), [1,1,nsample,1])
if points is not None:
grouped_points = group_point(points, idx)
if use_xyz:
grouped_points = tf.concat([grouped_points, grouped_xyz], axis=-1)
else:
grouped_points = grouped_xyz
if use_nchw: grouped_points = tf.transpose(grouped_points, [0,3,1,2])
for j,num_out_channel in enumerate(mlp_list):
grouped_points = tf_util.conv2d(grouped_points, num_out_channel, [1,1],
padding='VALID', stride=[1,1], bn=bn, is_training=is_training,
scope='conv%d_%d'%(i,j), bn_decay=bn_decay)
if use_nchw: grouped_points = tf.transpose(grouped_points, [0,2,3,1])
new_points = tf.reduce_max(grouped_points, axis=[2])
new_points_list.append(new_points)
new_points_concat = tf.concat(new_points_list, axis=-1)
return new_xyz, new_points_concat与SSG中不同,MSG中的radius和nsample不再是一个标量,而是一个数组,即存在一组不同的radius和nsample配置。也就是说,对输入的点云,我们有多种不同的分组方式,每次分组时,其子集的大小和稠密程度都有所不同,也即感知域不同。
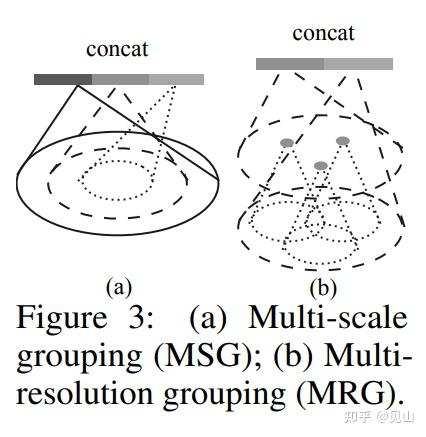
在实现上也很好理解,给定一个radius和与之相对应的nsample,其实就是一次SSG。也就是说做一次MSG等价于做多次SSG,每次SSG的输入点云和分组的组数是相同的,但radius和nsample以及相应的PointNet配置是不同的。
我们前面提到,SSG的pointnet_sa_module的输出:包括分组后的每个点的位置/每个组的中心(new_xyz),分组后的每个点的特征向量(new_points)。MSG的输出也可以由此得到:
- 因为分组时寻找子集中心的算法都是FPS,所以多次SSG得到的分组中心也都是相同的;
- 分组后的特征向量的尺寸为batch_size * npoint * channel,这里batch_size * npoint对每次SSG都是一样的,而channel在每次SSG中是不一样的,而每次SSG提取出的信息我们都希望传递到下一层,所以我们在channel这个维度将每次SSG的结果进行拼接,最后MSG输出的分组后特征向量的尺寸为batch_size * npoint * ∑channel。
以上就是Classification所使用的SSG和MSG的代码分析。
对于Semantic Segmantation来说,需要得到每个原始点的label,因此除了基于pointnet_sa_module的信息聚合以外,还需要将全局的结构信息层层回传。
def get_model(point_cloud, is_training, num_class, bn_decay=None):
""" Semantic segmentation PointNet, input is BxNx3, output Bxnum_class """
batch_size = point_cloud.get_shape()[0].value
num_point = point_cloud.get_shape()[1].value
end_points = {}
l0_xyz = point_cloud
l0_points = None
end_points['l0_xyz'] = l0_xyz
# Layer 1
l1_xyz, l1_points, l1_indices = pointnet_sa_module(l0_xyz, l0_points, npoint=1024, radius=0.1, nsample=32, mlp=[32,32,64], mlp2=None, group_all=False, is_training=is_training, bn_decay=bn_decay, scope='layer1')
l2_xyz, l2_points, l2_indices = pointnet_sa_module(l1_xyz, l1_points, npoint=256, radius=0.2, nsample=32, mlp=[64,64,128], mlp2=None, group_all=False, is_training=is_training, bn_decay=bn_decay, scope='layer2')
l3_xyz, l3_points, l3_indices = pointnet_sa_module(l2_xyz, l2_points, npoint=64, radius=0.4, nsample=32, mlp=[128,128,256], mlp2=None, group_all=False, is_training=is_training, bn_decay=bn_decay, scope='layer3')
l4_xyz, l4_points, l4_indices = pointnet_sa_module(l3_xyz, l3_points, npoint=16, radius=0.8, nsample=32, mlp=[256,256,512], mlp2=None, group_all=False, is_training=is_training, bn_decay=bn_decay, scope='layer4')
# Feature Propagation layers
l3_points = pointnet_fp_module(l3_xyz, l4_xyz, l3_points, l4_points, [256,256], is_training, bn_decay, scope='fa_layer1')
l2_points = pointnet_fp_module(l2_xyz, l3_xyz, l2_points, l3_points, [256,256], is_training, bn_decay, scope='fa_layer2')
l1_points = pointnet_fp_module(l1_xyz, l2_xyz, l1_points, l2_points, [256,128], is_training, bn_decay, scope='fa_layer3')
l0_points = pointnet_fp_module(l0_xyz, l1_xyz, l0_points, l1_points, [128,128,128], is_training, bn_decay, scope='fa_layer4')
# FC layers
net = tf_util.conv1d(l0_points, 128, 1, padding='VALID', bn=True, is_training=is_training, scope='fc1', bn_decay=bn_decay)
end_points['feats'] = net
net = tf_util.dropout(net, keep_prob=0.5, is_training=is_training, scope='dp1')
net = tf_util.conv1d(net, num_class, 1, padding='VALID', activation_fn=None, scope='fc2')
return net, end_points从代码上来看,Semantic Segmantation的网络结构也十分清晰:
- 用4层pointnet_sa_module将原始点云信息聚合为16个长度为512的特征向量;
- 用4层pointnet_fp_module将上述聚合信息回传到每个原始点的128维特征向量上;
- 用2层1维卷积将每个点的128维特征向量变换成类别数的One-Hot编码。
这里的pointnet_sa_module我们已经分析过,而pointnet_fp_module则是为了实现信息回传的模块。假设在多层的pointnet_sa_module中,我们将第2层输出的n2个点(每个点有3维位置和长度为d2的特征向量),输入到第3层,聚合为n3个分组即n3个点(每个点有3维位置和长度为d3的特征向量),这里n3<n2。
通过降采样正向聚合信息的思路很好理解,那么如何反向扩散信息呢?一个直观的思路是插值。
We achieve feature propagation by interpolating feature values f of N_l points at coordinates of the N_l−1 points. Among the many choices for interpolation, we use inverse distance weighted average based on k nearest neighbors (as in Eq. 2, in default we use p = 2, k = 3). The interpolated features on N_l−1 points are then concatenated with skip linked point features from the set abstraction level. 论文中具体指出使用了基于k近邻的使用反距离作为权重的加权平均插值。
为了便于理解,我们将上述第2层的n2个点称为下层点,将第3层的n3个点称为上层点。
想将n3个上层点的信息扩散到n2个下层点上,对于每个下层点,我们找出k个空间上最接近的上层点,我们认为这些上层点的信息包含了足够的关于这个下层点的信息。
拿到这k个最接近的上层点后,我们希望将他们进行加权平均来恢复这个下层点的信息,很自然的,如果某个上层点很接近这个下层点,那么我们希望它对最后的结果的贡献度大一些,即我们希望这个权重和上层点与下层点的距离直接相关,且与距离成单调减/相反变化的关系。

结合公式很好理解,这里d(x, x_i)表示下层点x和上层点x_i之间的距离,f_i_(j)表示和下层点j最接近的第i个上层点的特征向量。论文中说默认参数是k=3, p=2,代码中也是这样设置的。
def pointnet_fp_module(xyz1, xyz2, points1, points2, mlp, is_training, bn_decay, scope, bn=True):
&#39;&#39;&#39; PointNet Feature Propogation (FP) Module
Input:
xyz1: (batch_size, ndataset1, 3) TF tensor
xyz2: (batch_size, ndataset2, 3) TF tensor, sparser than xyz1
points1: (batch_size, ndataset1, nchannel1) TF tensor
points2: (batch_size, ndataset2, nchannel2) TF tensor
mlp: list of int32 -- output size for MLP on each point
Return:
new_points: (batch_size, ndataset1, mlp[-1]) TF tensor
&#39;&#39;&#39;
with tf.variable_scope(scope) as sc:
dist, idx = three_nn(xyz1, xyz2)
dist = tf.maximum(dist, 1e-10)
norm = tf.reduce_sum((1.0/dist),axis=2,keep_dims=True)
norm = tf.tile(norm,[1,1,3])
weight = (1.0/dist) / norm
interpolated_points = three_interpolate(points2, idx, weight)
if points1 is not None:
new_points1 = tf.concat(axis=2, values=[interpolated_points, points1]) # B,ndataset1,nchannel1+nchannel2
else:
new_points1 = interpolated_points
new_points1 = tf.expand_dims(new_points1, 2)
for i, num_out_channel in enumerate(mlp):
new_points1 = tf_util.conv2d(new_points1, num_out_channel, [1,1],
padding=&#39;VALID&#39;, stride=[1,1],
bn=bn, is_training=is_training,
scope=&#39;conv_%d&#39;%(i), bn_decay=bn_decay)
new_points1 = tf.squeeze(new_points1, [2]) # B,ndataset1,mlp[-1]
return new_points1这里的three_nn即是对每个下层点求最近的3个上层点的距离和索引的函数,这里的three_nn是封装的C++函数,从C++源码中可以看到dist计算的是平方距离,也就是对应了论文中说的p=2。
通过上述加权平均插值,我们将第3层的n3个点的特征向量扩散到了第2层的n2个点,扩散后的特征向量由于是第3层的特征向量的加权平均, 因而保留了相同的长度d3。
注意到本来第2层的pointnet_sa_module就为每个点聚合出了长度为d2的特征向量,将这两个特征向量进行拼接,再通过一个MLP进行组合,即完成了一次完整的信息回传扩散。
在层级化的Set Abstraction中,从低到高,特征向量携带的信息从局部扩展到全局,因此上述拼接的两个特征向量中,d3包含了更全局的信息,而d2则更关注于局部信息,两者相结合,共同用来确定这个层级上每个点的特征信息。
Part Segmentation的逻辑和Semantic Segmantation类似,不再赘述。
至此,PointNet++代码结构及逻辑分析完毕。 |
|